LLMs
Introduction
A large language model is a computer program that learns and generates human-like language using a transformer architecture trained on vast training data.
Large Language Models (LLMs) are foundational machine learning models that use deep learning algorithms to process and understand natural language. These models are trained on massive amounts of text data to learn patterns and entity relationships in the language. LLMs can perform many types of language tasks, such as translating languages, analyzing sentiments, chatbot conversations, and more. They can understand complex textual data, identify entities and relationships between them, and generate new text that is coherent and grammatically accurate, making them ideal for sentiment analysis.
What is a Large Language Model (LLM)?
A large language model is an advanced type of language model that is trained using deep learning techniques on massive amounts of text data. These models are capable of generating human-like text and performing various natural language processing tasks.
In contrast, the definition of a language model refers to the concept of assigning probabilities to sequences of words, based on the analysis of text corpora. A language model can be of varying complexity, from simple n-gram models to more sophisticated neural network models. However, the term “large language model” usually refers to models that use deep learning techniques and have a large number of parameters, which can range from millions to billions. These AI models can capture complex patterns in language and produce text that is often indistinguishable from that written by humans.
What are LLMs used for?
Large language models (LLMs) are finding application in a wide range of tasks that involve understanding and processing language. Here are some of the common uses:
Content creation and communication: LLMs can be used to generate different creative text formats, like poems, code, scripts, musical pieces, emails, and letters. They can also be used to summarize information, translate languages, and answer your questions in an informative way.
Analysis and insights: LLMs are capable of analyzing massive amounts of text data to identify patterns and trends. This can be useful for tasks like market research, competitor analysis, and legal document review.
Education and training: LLMs can be used to create personalized learning experiences and provide feedback to students. They can also be used to develop chatbots that can answer student questions and provide support.pen_spark
How a Large Language Model (LLM) is Built?
A large-scale transformer model known as a “large language model” is typically too massive to run on a single computer and is, therefore, provided as a service over an API or web interface. These models are trained on vast amounts of text data from sources such as books, articles, websites, and numerous other forms of written content. By analyzing the statistical relationships between words, phrases, and sentences through this training process, the models can generate coherent and contextually relevant responses to prompts or queries. Also, Fine-tuning these models involves training them on specific datasets to adapt them for particular applications, improving their effectiveness and accuracy.
ChatGPT’s GPT-3, a large language model, was trained on massive amounts of internet text data, allowing it to understand various languages and possess knowledge of diverse topics. As a result, it can produce text in multiple styles. While its capabilities, including translation, text summarization, and question-answering, may seem impressive, they are not surprising, given that these functions operate using special “grammars” that match up with prompts.
How do LLMs work?
Large language models like GPT-3 (Generative Pre-trained Transformer 3) work based on a transformer architecture. Here’s a simplified explanation of how they Work:
Learning from Lots of Text: These models start by reading a massive amount of text from the internet. It’s like learning from a giant library of information.
Innovative Architecture: They use a unique structure called a transformer, which helps them understand and remember lots of information.
Breaking Down Words: They look at sentences in smaller parts, like breaking words into pieces. This helps them work with language more efficiently.
Understanding Words in Sentences: Unlike simple programs, these models understand individual words and how words relate to each other in a sentence. They get the whole picture.
Getting Specialized: After the general learning, they can be trained more on specific tasks to get good at certain things, like answering questions or writing about particular subjects.
Doing Tasks: When you give them a prompt (a question or instruction), they use what they’ve learned to respond. It’s like having an intelligent assistant that can understand and generate text.
Difference Between Large Language Models and Generative AI
Scope
Generative AI encompasses a broad range of technologies and techniques aimed at generating or creating new content, including text, images, or other forms of data.
Large Language Models are a specific type of AI that primarily focus on processing and generating human language.
Specialization
It covers various domains, including text, image, and data generation, with a focus on creating novel and diverse outputs.
LLMs are specialized in handling language-related tasks, such as language translation, text generation, question answering, and language-based understanding.
Tools and Techniques
Generative AI employs a range of tools such as GANs (Generative Adversarial Networks), VAEs (Variational Autoencoders), and evolutionary algorithms to create content.
Large Language Models typically utilize transformer-based architectures, large-scale training data, and advanced language modeling techniques to process and generate human-like language.
Role
Generative AI acts as a powerful tool for creating new content, augmenting existing data, and enabling innovative applications in various fields.
LLMs are designed to excel in language-related tasks, providing accurate and coherent responses, translations, or language-based insights.
Evolution
Generative AI continues to evolve, incorporating new techniques and advancing the state-of-the-art in content generation.
Large Language Models are constantly improving, with a focus on handling more complex language tasks, understanding nuances, and generating more human-like responses.
So, generative AI is the whole playground, and LLMs are the language experts in that playground.
General Architecture
The architecture of Large Language Model primarily consists of multiple layers of neural networks, like recurrent layers, feedforward layers, embedding layers, and attention layers. These layers work together to process the input text and generate output predictions.
The embedding layer converts each word in the input text into a high-dimensional vector representation. These embeddings capture semantic and syntactic information about the words and help the model to understand the context.
The feedforward layers of Large Language Models have multiple fully connected layers that apply nonlinear transformations to the input embeddings. These layers help the model learn higher-level abstractions from the input text.
The recurrent layers of LLMs are designed to interpret information from the input text in sequence. These layers maintain a hidden state that is updated at each time step, allowing the model to capture the dependencies between words in a sentence.
The attention mechanism is another important part of LLMs, which allows the model to focus selectively on different parts of the input text. This self-attention helps the model attend to the input text’s most relevant parts and generate more accurate predictions.
Examples of LLMs
Let’s take a look at some popular large language models(LLM):
BERT (Bidirectional Encoder Representations from Transformers) – Developed by Google, BERT is another popular LLM that has been trained on a massive corpus of text data. It can understand the context of a sentence and generate meaningful responses to questions.
XLNet – This LLM developed by Carnegie Mellon University and Google uses a novel approach to language modeling called “permutation language modeling.” It has achieved state-of-the-art performance on language tasks, including language generation and question answering.
T5 (Text-to-Text Transfer Transformer) – T5, developed by Google, is trained on a variety of language tasks and can perform text-to-text transformations, like translating text to another language, creating a summary, and question answering.
RoBERTa (Robustly Optimized BERT Pretraining Approach) – Developed by Facebook AI Research, RoBERTa is an improved BERT version that performs better on several language tasks.
Open Source Large Language Model(LLM)
The availability of open-source LLMs has revolutionized the field of natural language processing, making it easier for researchers, developers, and businesses to build applications that leverage the power of these models to build products at scale for free. Examples are Llama 3, Mistral, Bloom.
Llama 3 8B
Llama 3 8B is Meta’s 8-billion parameter language model that was released in April 2024. It is an improvement to the previous generation, Llama 2, with the data training set being seven times as large, with a more significant emphasis on code. The model is well-suited for various use cases, such as text summarization and classification, sentiment analysis, and language translation.
Mistral 7B
Mistral 7B is a dense transformer model that strikes a balance between performance and cost efficiency. Released in September 2023 by Mistral.ai, Mistral 7B has been a popular choice for those seeking a smaller, more affordable language model. Use cases include text summarization and structuration, question-answering, and code completion.
It is the first multilingual Large Language Model (LLM) trained in complete transparency by the largest collaboration of AI researchers ever involved in a single research project. With its 176 billion parameters (larger than OpenAI’s GPT-3), BLOOM can generate text in 46 natural languages and 13 programming languages. It is trained on 1.6TB of text data, 320 times the complete works of Shakespeare.
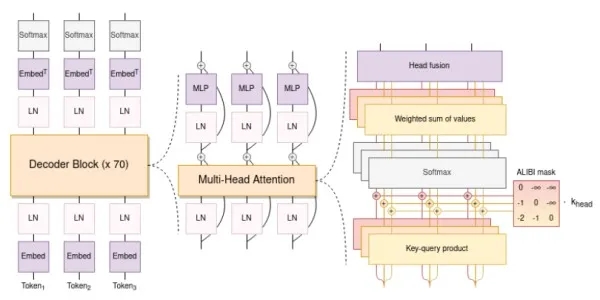
The architecture of BLOOM shares similarities with GPT3 (auto-regressive model for next token prediction), but has been trained in 46 different languages and 13 programming languages. It consists of a decoder-only architecture with several embedding layers and multi-headed attention layers.
Future Implications of LLMs
In recent years, there has been specific interest in large language model (LLMs) like GPT-3, and chatbots like ChatGPT, which can generate natural language text that has very little difference from that written by humans. These foundation models have seen a breakthrough in the field of artificial intelligence (AI). While LLMs have seen a breakthrough in the field of artificial intelligence (AI), there are concerns about their impact on job markets, communication, and society.
One major concern about LLMs is their potential to disrupt job markets. Large Language Model, with time, will be able to perform tasks by replacing humans like legal documents and drafts, customer support chatbots, writing news blogs, etc. This could lead to job losses for those whose work can be easily automated.
However, it is important to note that LLMs are not a replacement for human workers. They are simply a tool that can help people to be more productive and efficient in their work through automation. While some jobs may be automated, new jobs will also be created as a result of the increased efficiency and productivity enabled by LLMs. For example, businesses may be able to create new products or services that were previously too time-consuming or expensive to develop. By leveraging LLMs, they can optimize processes and improve efficiency, leading to innovation and growth.
LLMs have the potential to impact society in several ways. For example, LLMs could be used to create personalized education or healthcare plans, leading to better patient and student outcomes. LLMs can be used to help businesses and governments make better decisions by analyzing large amounts of data and generating insights.
Last updated